The representative weather data
The observational set
Processing techniques
Meteorological re-analysis and spatialization
The meteorological reanalysis method (or retrospective analysis) is the most advanced scientific technique for reconstructing past data. By combining numerical atmospheric simulation models with data assimilation systems used in forecasting, homogeneous datasets describing the past states of the atmosphere over a given area are produced.
Reanalysis incorporates data from different sources of measurement systems and integrates it into a digital meteorological grid. This grid uses spatialisation algorithms to reconstruct the state of the atmosphere in terms of its forecast variables at a certain point in time and in a continuous fashion over the area.
Nowcasting
The nowcasting methodology is used to produce very short-term forecasts (0 to 3 hours). It is characterised by the extensive integration of the latest data collected by the monitoring networks (on site and remote), into forecasting models formulated frequently, in order to obtain forecasts that are always up to date and consistent with the latest observations.
Nowcasting guarantees adequate coverage of the spatial-temporal window for which the forecast analysis is carried out. It is used for very short-term forecasting of rainfall and of those meteorological parameters (such as temperature, cloud cover, wind speed and direction, and solar radiation) that are significant for organisations managing the feed-in of energy from renewable energy plants.
Multimodel ensemble predictions
Postprocessing & Neural Networks
Quality control and data validation
Virtual domains, grids and stations
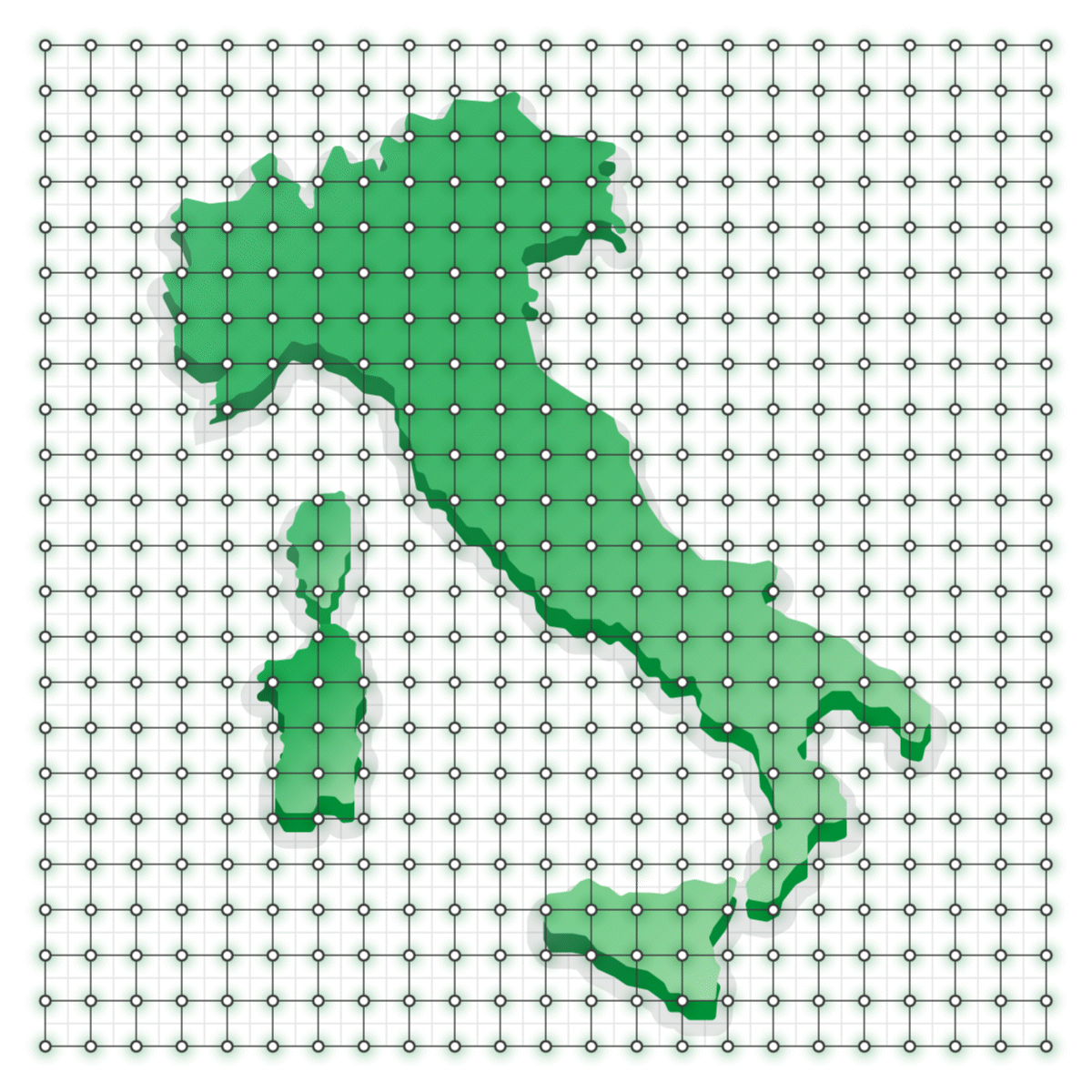
Hypermeteo’s customers
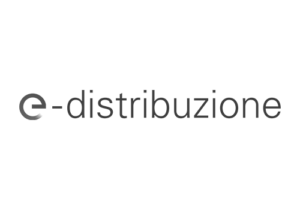
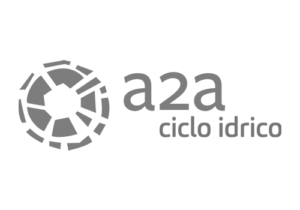
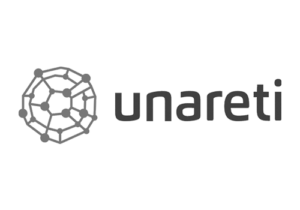
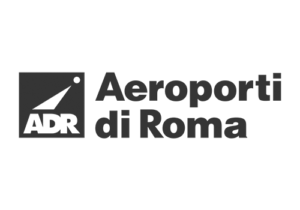